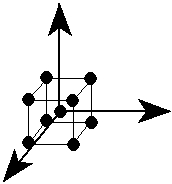
|
Mathews Malnar and Bailey,
Inc.
Quality
engineering, applied statistical consulting,
and training services for R&D, product, process,
and manufacturing engineering organizations. |
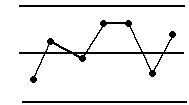
|
The Quality Engineers Network (QEN) is
sponsored by Geauga
Growth Partnership. Meeting announcements are in
reverse order with the most recent meeting at the top. All meetings
are free and everyone is welcome to present or to
recommend a topic. Please e-mail me at paul@mmbstatistical to be
added to the mailing list.
Use these meetings to earn recertification units (RUs) for your
ASQ certifications.
Book Review: Wayne Taylor, Statistical Procedures for the
Medical Device Industry, 8 August 2025, 7:30-9:00AM, by Zoom
One of the difficulties in learning applied statistical methods
and quality engineering is that the information you need to learn
is scattered over many text books, journals, industry and
regulatory standards, and on-line sources. Even after culling my
own library of reference materials I still have over 100 textbooks
that I can't part with. There are a few books that stand out, like
Nancy Tague's The Quality Toolbox, Juran's Quality
Control Handbook, Doug Montgomery's Statistical Quality
Control, and others. (Ask me for a recommendation if there's
a specific topic that you're interested in.) One of the very
impressive recent additions that I highly recommend is Wayne
Taylor's Statistical Procedures for the Medical Device
Industry. Even though the title of the book calls out the
medical device field the material in the book is of general
interest with coverage of acceptance sampling, SPC, designed
experiments, reliability, etc. The book is not cheap - it's about
$300 for the hard-copy and/or e-copy - but considering its content
it's a wise investment. When I purchased the book I bought it in
both hard- and e-copies so for this month's QEN meeting I'll lead
you through my e-copy so you can see what the book provides. You
can learn more about the book here.
I don't mean this QEN session to be an in-depth investigation into
any of the methods in Taylor's book. My general intent for QEN is
to stick to more basic topics and leave the more advanced topics
to QMN; however, someone getting started in quality engineering
statistics and quality engineering or just trying to fill the gaps
in their knowledge would be wise to consider investing in this
book. The book is very expensive but it's something that most
organizations should buy and make available to their staff.
Which Interval Do I Need: Confidence, Prediction, or
Tolerance?, 11
April 2025, 7:30-9:00AM, by Zoom
Statistical software like MINITAB can be an amazing tool
but it can also present a bewildering collection of methods that
look so much alike that it can be difficult to determine which
ones are interchangeable and which ones are different. One such
collection of methods is statistical intervals including
confidence intervals, prediction intervals, and tolerance
intervals. At this month's QEN meeting we'll discuss the
calculation, use, and interpretation of each type of statistical
interval. If you have any examples or data that you would like to
share for this discussion, please send them to me (Paul) with a
short description of what you're trying to do.
The Blake and Mouton Leader/Follower
Model, 7:30-9:00AM, 14 March 2025, by Zoom
One of the most important things we have to learn in the practice
of applied statistics is how to deal with people who are
statistically naive. I was recently working with one of my
customers who was in this situation. He was trying to support one
of his suppliers to solve a quality problem and the supplier was
naive of the methods that were required. My customer was
frustrated at the schedule delays and the push-back he was
receiving from his supplier - who was effectively resisting his
recommendations to resolve the problem. As we were discussing
available strategies for him to deal with his customer, I realized
I was invoking methods I learned in a GE course long ago. The
impact of that training on me was profound and I subconsciously
use the methods I learned when dealing with pretty much everyone
including my customers, bosses, coworkers, and students.
Way back when I worked for GE they put us through a lot of
training. One of the many great courses that I got to take was
their two week long Project Leadership Course. One of the
fascinating topics they taught us was the Blake and Mouton
leader/follower model. Blake and Mouton were management theorists
who were trying to determine what leadership styles were most
effective. To answer that question, they had to characterize
leader and follower behaviors. In the simplest implementation of
their system, they created a two-dimensional model for leaders
based on 1) task-oriented behavior and 2) relationship-oriented
behavior. For the followers they created a two-dimensional model
based on 1) ability and 2) motivation. When they collected data
studying the relationship between leader and follower behaviors
they were able to identify an optimal (in terms of overall leader
and follower system performance) leadership strategy for managers.
Many of the stories that I tell in classes and at meetings come
from learnings from the Blake and Mouton model. I'll talk about
these and many other cases at this month's meeting.
Deming's Funnel Experiment, 7:30-9:00AM,
14 February 2025, by Zoom
Deming's funnel experiment was one of the exercises he performed
during his famous three day seminars. The purpose of the
experiment is to show the effect of tampering with a process, i.e.
the effect of attempting to control a process when it is only
subject to random/common cause variation. The experiment can be
done with Deming's original hardware (a funnel supported by a ring
stand and marbles), by dropping playing cards, or by computer
simulation. We're meeting remotely so we'll do this by computer
simulation in MINITAB.
Deming considered four process control rules in the experiment:
1) Hands off, i.e. don't attempt to
control the process
2) Correct the funnel position relative to the
last position
3) Correct the funnel position relative to the
target
4) Move the funnel to the last trial's position,
i.e. make the next part like the previous one
These simple rules capture some of the most common
mistakes people make when trying to control their processes. The
exercise helps demonstrate these mistakes so you can recognize and
eliminate them in your own processes.
IT'S A TRAP! (Box-Cox and Johnson Transforms), 7:30-9:00AM, 13 December 2024, by
Zoom
A constant problem that we all have is choosing an
appropriate distribution model for a problematic data set. MINITAB
has the fabulous Stat> Quality Tools> Individual
Distribution Identification method which fits 16 different
distribution models to your data, including the Box-Cox and
Johnson transforms; however, during training, I always warn
against liberal use of these methods - especially the Box-Cox and
Johnson transforms - because I think they are a trap. John von
Neumann concisely explained the problem: "Give me three
parameters and I can fit an elephant. Give me four parameters
and I can make him wiggle his trunk." When you attempt so
many distribution models something is going to look good, but
usually the best fit won't make any sense, especially when there
are probably other problems in the data. So as appealing as these
distribution fitting tools are, I think their use reeks of
desperation and most often leads to a one-time solution that will
probably betray you in the future. At this month's QEN meeting
we'll look at some purposely (via simulation) problematic data
sets to see what distribution models fit and if there was any
chance to detect and avoid the problem.
Which Analysis to Choose: Confidence Interval or Hypothesis
Test?, 7:30-9:00AM, 8 November 2024 via Zoom
We know that point estimates like the sample mean and standard
deviation are insufficient for making data-based decisions because
they don't take estimation precision into account. Proper
data-based decision making requires the use of an inferential
statistics method - either a confidence interval or a hypothesis
test. The choice between the two methods of analysis has been made
for us for many common applications, e.g. SPC, DOE, etc.; however,
the choice may not be so clear in other cases. At this month's QEN
meeting we will discuss the factors that should be considered when
trying to choose between a confidence interval and a hypothesis
test for the analysis of a planned experiment.
Applications of Chat GPT to Quality
Engineering Problems, 7:30-9:00AM, 11 October 2024, by Zoom
At our September QEN meeting Sergei Ivanov of FoundAItion AI
described the basics of using of Chat GPT for general problem
solving. (E-mail me for Sergei's contact information if you want
to follow up with him.) I (Paul) have been experimenting with the
use of Chat GPT in more specific quality engineering applications
including SPC, acceptance sampling, formulation of hypothesis test
statements, sample size calculations, etc. At this month's QEN
meeting I will present some of my results. We should have time to
consider other problems if you have something in mind that would
be interesting.
Applications of Chat GPT in Manufacturing
Quality Engineering, 13 September, 7:30-9:00AM, by Zoom
Join us for an engaging presentation that explores the
transformative capabilities of Chat GPT in Manufacturing Quality
Engineering. This session will cover the basics of prompt
engineering and demonstrate practical applications of Chat GPT in
solving common engineering problems. Attendees will learn how to
use Chat GPT to diagnose quality control issues, generate
technical reports, draft internal memos, plan projects, and assist
in research and analysis. Through hands-on exercises, we will show
how AI can enhance efficiency and accuracy in manufacturing
processes. Discover how to leverage Chat GPT to improve your
workflow and achieve quality excellence.
Nonstandard SPC Charts, 9
August 2024, 7:30-9:00AM, by Zoom
At our last two QEN meetings we discussed the
Shewhart family of SPC charts. At this month's meeting we will
consider some nonstandard charts including
- Taguchi's Loss Function chart
- Shewhart charts for unusual statistics
- median and midrange charts
- charts for order statistics, e.g. min, max,
...
- Acceptance control charts
- Time-weighted charts
- moving average
- EWMA
- CUSUM
- Groups charts for multi-stream processes
- Multivariate charts
This is a lot of material so we'll only have time
to do superficial coverage of these methods. Most of them are
supported in MINITAB.
Introduction to Statistical Process Control (SPC)
Part 2, 12 July 2024, 7:30-9:00AM, by Zoom
At our June QEN meeting we discussed:
- Taguchi's Loss Function as a motivator for
using SPC charts to reduce lost value by controlling a
process's location and variation
- How control charts help us distinguish between
common cause/noise variation and special cause/assignable
cause variation which require different process management
actions
- The distinction between statistical process control
which falls in the prevention (i.e. the best) quality cost
category and statistical process documentation which
is at best an appraisal cost and at worst a failure cost.
We discussed the most common form of control
charts, the Shewhart charts, including:
- Defectives (np) charts for defective counts
with constant sample size
- Proportion defective (p) charts for defective
counts with variable sample size
- Defects (c) charts for defect counts with
constant sample size
- Defect per unit (u) charts for defect counts
with variable sample size
- Xbar and R charts for variables data with
subgroup sample size n > 1
- Individual and Moving Range (IMR) charts for
variable data with subgroup sample size n = 1
We also talked about how to configure these
charts in MINITAB, how to specify the observations to use for
calculating control limits, and how to set up and interpret run
rules to detect out-of-control events.
At this month's QEN meeting we will continue our
discussion of SPC charts including:
- Run rules: How they work, how they are
designed, and the Western Electric rules
- The risks associated with using too many run
rules and too many control charts
- Details of center line and control limit
calculations
- SPC chart lifetime
An Introduction to SPC with Implementation in MINITAB, 14 June
2024, 7:30-9:00AM, by Zoom
At the request of a local company we are going to discuss the
basics of Statistical Process Control (SPC) at this month's QEN
meeting. We will begin with a discussion of the different types of
process variation (common cause, special/assignable cause,
structural, and tampering) and the different strategies to manage
them. Then we will move on to the use of SPC control chart methods
to distinguish between common cause variation (which requires no
action) and special/assignable cause variation (which requires
action). We will discuss the design and operation of the most
common Shewhart control charts including design strategies for
choosing samples, the calculation of control limits, the use of
run rules, the risks of using too many run rules or keeping too
many charts, and the implementation of these charts using MINITAB.
The Kano Model for Classifying Process
Output Variables, 10 May 2024, 7:30-9:00AM, by Zoom
You guys know that I like to use an Input-Process-Output (IPO)
diagram to document all of a process's process input variables
(PIV) and process output variables (POV). The next step in
understanding the process is classifying its POVs as Critical to
Quality (CTQ), Key Process Output Variables (KPOV), and ordinary
Process Output Variables (POV). This classification scheme comes
from Six Sigma and works very well; however, I think that the
distinction between the CTQs and KPOVs can be a bit murky. I
usually describe the CTQs as characteristics that the customer
specifically requests and the KPOVs as other characteristics that
they assume will be present but don't know to ask for. I took
these definitions from the Kano Model, which I think does a better
job of classifying the POVs than the Six Sigma method does. We'll
look at the Kano Model at this month's QEN meeting.
Kano Model: https://en.wikipedia.org/wiki/Kano_model
Interpreting the Results of a Medical Diagnostic Test, 9 February 2024, 7:30-9:00AM, by Zoom
There is a famous biomedical statistics problem that I use
in homework assignments that was originally published by Edler and
then followed up by Gerd Gigerenzer. The problem involves a cancer
diagnostic test and the counterintuitive implications of a
positive test result. ("Positive" here means positive for cancer.)
In Edler's original publication he showed that many doctors
incorrectly interpreted the result of the test. Gigerenzer showed
that doctors could be taught to use a simple analysis method for
analyzing the problem. The formal analysis of the problem uses
Bayes's Theorem; however, Gigerenzer's approach only requires the
use of a simple Venn diagram. We'll look at this problem and its
variations at this month's meeting. If you don't have time to join
us you can watch the brilliant presentation of the problem by
Grant Sanderson (3 Blue 1 Brown) on Youtube here.
Analysis of Means, 12 January 2024, 7:30-9:00AM, via Zoom
The go-to method for testing for differences between treatment
groups means in one-way or multi-way classification designs is the
Analysis of Variance (ANOVA) method. An alternative but lesser
known family of methods is the Analysis of Means (ANOM). ANOM has
the advantage of presenting its results in a graphical form very
similar to a control chart which can be useful for presenting to a
statistically naive audience. At this month's QEN meeting we will
consider the ANOM methods for variables data, proportions, and
counts and MINITAB's implementation of them in the Stat>
ANOVA> Analysis of Means menu.
Variable Transforms, 10 November
2023, 7:30-9:00AM, by Zoom
Most statistical analysis methods that test a characteristic of a
distribution (e.g. mean, standard deviation, or distribution
shape) make assumptions about the behavior of the data. These
assumptions must be tested before the results of the chosen
analysis method can be accepted. When an assumption is violated,
then the analysis method's results can't be trusted and a
corrective action must be applied or a different analysis method
must be used. Among the most effective corrective actions is the
application of a variable transform. For example, a violation of
the normality or homoscedasticity (i.e. equal standard deviations)
assumption can often be resolved by taking the square root,
square, reciprocal, or log of the original data. At this month's
QEN meeting we will look at how to recognize when an assumption of
a statistical analysis method is violated and how to resolve the
problem using an appropriate variable transform.
Confession Time: Things I've Done That Will Send Me to
Statistics Hell, 13 October 2023, 7:30-9:00AM, by Zoom
Whether it's happened out of naivite, laziness, or desperation,
I'm sure that we've all compromised "best practice" in some aspect
of our statistical work. It's confession time: Let's share some of
the poor choices we made, how we came to or why we were forced to
make them, and how we could do better. It's probably too late to
keep you out of statistics hell but this may help you avoid moving
further up in line.
Manipulating Data in MINITAB, 8
September 2023, 7:30-9:00AM, by Zoom
Most of us use MINITAB for our statistical analysis needs but
often format our data for analysis in Excel and then copy the
Excel worksheet over into MINITAB. MINITAB has its own rich tool
set for data manipulation including many features missing from
base Excel. At this month's QEN meeting we will look at MINITAB's
many tools for data manipulation.
Specifying Success Criteria for
Attribute GR&R Studies, 11 August 2023, 7:30-9:00AM, by
Zoom
GR&R attribute studies require a completely different set of
performance metrics from their variables data siblings because of the pass/fail nature of their
inspection results. If you look at the output from
MINITAB's analysis of an attribute R&R study there is a
bewildering collection of statistics - some which might make some
sense and others which are completely cryptic. At this month's QEN
meeting we will talk about what these different performance
metrics are used for and which of them present the best
opportunities to define attribute R&R study success criteria.
Assessing Agreement Between Two Measurement Systems with the
Bland-Altman Method, 9 June 2023, 7:30-9:00AM, by Zoom
Some measurement systems can be difficult to use, take too
long to perform, or are too expensive. In such cases, it is
natural that an measurement alternative system be considered that
addresses these issues; however, how do we compare the old and new
measurement systems to quantify their agreement? The first
analysis method that comes to mind is a simple correlation
analysis: Measure many units spanning a range of measurement
values using both measurement systems and calculate the
correlation coefficient between paired observations; however, the
correlation coefficient can be made arbitrarily large even when
the agreement between the two measurements is poor by choosing a
very wide range of values. The preferred method of analysis is
Tukey's mean-different plot which was popularized by Bland and
Altman. The Bland-Altman method is superior to the correlation
method because it addresses issues of bias and scaling in absolute
terms.
Experiment Protocol Documents, 12 May 2023, 7:30-9:00AM, by
Zoom
As a consultant I've seen a wide range of practices with respect
to how organizations plan and execute their experimental work.
Those that skimp on the early planning and preparation phases and
rush to build their experiments tend to experience more failures
that are discovered late in the process when the consequences of
lost time, wasted resources, slipped schedules, and aggravated
managers is the most traumatic. Other organizations, such as those
that are highly regulated, tend to use highly structured formal
procedures that include much more up-front planning. The key
document that distinguishes the two groups, the document that the
highly regulated group depends on and the skimpers lack, is often
referred to as an experiment protocol. From what I've seen,
experiments run under protocol tend to go more smoothly and have
better endings than those that aren't. So at this month's meeting
we'll discuss the content of an experiment protocol document and
develop a protocol template that we can all share for managing our
own experimental processes.
Design and Operation of Variables
Sampling Plans for Defectives, 7:30-9:00AM, Friday, 14 April
2023, by Zoom
At last month's QEN meeting we discussed the calculation of and
application of normal tolerance intervals. A closely related and
well known alternative method is variables sampling plans (VSP)
for defectives. VSPs are defined in the same terms as attribute
sampling plans: By acceptable quality level (AQL) and rejectable
quality level (RQL) conditions where:
- AQL condition: The sampling plan should have a
high probability (e.g. 95%) of accepting lots with low
proportion defective (the AQL level)
- RQL condition: The sampling plan should have a
low probability (e.g. 10%) of accepting lots with high
proportion defective (the RQL level).
In an attribute sampling plan we draw a sample of
specified size n, inspect the sample and count the number of
defectives (D), and then accept the lot if D is less than or equal
to a critical acceptance number c. In a VSP the decision to accept
or reject a lot is based on a measurement response that is assumed
to be normally distributed. If the sample mean is far enough away
from the specification limits then the lot will be accepted and if
the sample mean is too close to a specification limit then the lot
will be rejected. The VSP's sample size n and critical distance k
are defined by the choice of AQL and RQL values. At this month's
meeting we will discuss the design and operation of VSPs by manual
means and using MINITAB.
Normal Tolerance
Intervals for Two or More Treatment Groups, 7:30-9:00AM, 10 March 2023, by
Zoom
Given measurement data from a sample, a normal
tolerance interval can be used to calculate an interval that
contains a specified proportion of a population with a
specified confidence level. Common applications for normal
tolerance intervals are:
- A design
engineer uses a normal tolerance interval to calculate
preliminary specification limits from available data.
- A
manufacturing engineer uses a normal tolerance interval to
show that his process is operating within specification
limits.
Normal tolerance
intervals are calculated from a sample's mean (xbar) and
standard deviation (s) and a factor (k1
or k2)
that accounts for the distribution of the population and the
estimation precision for the population mean and standard
deviation. Normal tolerance intervals have the form:
- UTL/LTL
= xbar +/- k2s for a two-sided tolerance
interval
- UTL =
xbar + k1s for a one-sided upper
tolerance interval
- LTL =
xbar - k1s
for a one-sided lower tolerance interval
where the k1
and k2
factors are functions of the coverage (i.e. the desired
fraction of the population in the interval), the confidence
level, and the sample size. k1
and k2
values are available in published tables and they are built
into MINITAB's Stat> Quality Tools> Tolerance
Intervals (Normal Distribution) method.
Although the normal tolerance interval method is fantastically
useful when dealing with a single population, it is very
common to have data that come from multiple treatment groups
with fixed levels. For example, a medical device might need to
operate under diverse orientation and environmental
conditions. In such cases, individual normal tolerance
intervals can be calculated for each unique treatment group;
however, when the device is expected to be robust to changes
in its operating conditions then all of the treatment groups
often have similar or related behavior so their information
can be pooled. This approach presents the opportunity to
reduce an experiment's overall sample size by combining the
information from all of the treatment groups. Although this
method of pooling information from two or more treatment
groups for a normal tolerance interval analysis is discussed
in the literature it is not well known and it is not
implemented in MINITAB. So at this month's QEN meeting we will
discuss how to construct normal tolerance intervals for two or
more treatment groups by combining their information into a
single analysis.
Outliers!, 7:30-9:00AM, 10 February 2023, by
Zoom
I've been struggling recently to help a customer analyze
his lab data. The analysis wouldn't be difficult except that
there are occasional outliers in the data sets. Sometimes there
are a few outliers that, together, look like they're from a long
tail of the distribution. In other cases the outliers look very
much like they come from a different population than the rest.
At this month's QEN meeting we will discuss methods for
detecting outliers, their possible causes, and the right and
wrong way to handle them in our analyses.
The Effect of Part Choice on Gage R&R Study Results, 7:30-9:00AM, 13 January 2023, by Zoom
The classic gage R&R study experiment design uses three
operators who each measure ten parts twice. In previous meetings
we've discussed why three operators isn't enough and why measuring
twice is sufficient. Modern guidance recommends at least seven
operators (Burdick, Borror, and Montgomery: Design and
Analysis of Gage R&R Studies). And while ten parts is
often sufficient, how parts are chosen for the study can have a
large effect on the results. The most common methods for choosing
parts for a gage R&R study are 1) Choose parts typical of the
process and 2) Choose parts that span the range of the tolerance
but these choices can give very different results. We'll take a
look at these choices and other possibilities at this month's
meeting.
Analysis of Censored Data, 7:30-9:00AM, 9 December 2022, by
Zoom
In most inspection or measurement operations we collect complete
data; that is, we collect an observation from each unit in a
sample and we use well known methods for analyzing the complete
data. However, there are situations in which it may be impossible
or impractical to collect an observation on some units in a
sample. Data of this type are said to be incomplete or censored
and require special analysis methods.
A customer recently asked me to help him analyze a data set. He
was performing a tensile test of the force required to pull a drug
vial from its mating adapter; however, sometimes during testing
the adapter got pulled out of the tensile tester's chuck instead.
This is an example of censored data: The drug vial/adapter
interface was stronger than the adapter/chuck interface so the
vial/adapter force is not known but the adapter/chuck force
provides a lower limit for its value. Proper analysis of these
data requires simultaneous analysis of all observations, including
those observations that have measured vial/adapter forces AND
those observations that were censored by the adapter/chuck force.
At this month's QEN meeting we'll talk about how to analyze
incomplete/censored data like this, how to get MINITAB to do the
work, and how to interpret the results.
Statistical Quick Tests, 7:30-9:00AM,
11 November 2022, by Zoom
We all know that most statistical tests involve some manual
calculation or require special software. The calculations aren't
too difficult to perform but they do take some time, resources,
perhaps a table or critical values, and of course remembering how
to perform the analysis. Alternatively, there are some well known
statistical "quick tests" that can be performed by a quick
inspection of an appropriate table or graph. These methods aren't
as powerful as others, but they have the benefit of being fast and
so easy that you can't prevent yourself from applying them given
the opportunity. This month we'll look at Tukey's Quick Test and
the Boxplot Slippage Tests for the two-sample location problem,
some other quick test methods, and some other closely related
methods.
Case Study: Analysis of Very
Noisy Serial Data, 7:30-9:00AM, 14 October
2022, by Zoom
I was recently helping network member Ralph L analyze some
interesting lab data. His experiment involves two treatment groups
- a test group and a control group - and he is trying to determine
if there is a difference between the means of the two groups. The
first complication is that the data are very noisy, covering a
very wide range of response values - about 4 orders of magnitude!
That problem is quickly dealt with using a log transform. The next
complication is that Ralph has serial data, i.e. he has collected
many observations periodically on the same samples and over a
considerable period of time. Serial data tend to be strongly
correlated so it would be incorrect to treat the observations as
if they were independent. That problem is dealt with by some
simple pre-processing of the data prior to performing the ultimate
two-sample test for a difference between the two treatment group
means. Ralph has volunteered to let us use his anonymized
experimental data for this discussion. If you want to get a jump
on understanding the analysis method check out this classic paper
on the topic https://www.ncbi.nlm.nih.gov/pmc/articles/PMC1662443/.
Editing Graphs in MINITAB, 7:30-9:00AM, 9 September 2022, by Zoom
In addition to its broad scope and depth in the statistical
methods topics, MINITAB software provides an equally broad and
deep set of graphical methods. MINITAB's default graphs are
satisfactory for most purposes, but when a MINITAB graph must be
modified to extract or highlight every piece of important
information MINITAB also provides fantastic graph editing and
customization tools. At this month's QEN meeting we will review
the graph design features that MINITAB exposes in its normal menus
and then we'll look at how to double click and right click your
way through customizing your MINITAB-produced graphs to make the
perfect graphs that you need.
Use Expectation Value to Evaluate and Compare
Business Process Models, 7:30-9:00AM, 12 August 2022, by Zoom.
The method of expectation values is introduced in statistics
courses to determine the parameters of a distribution; however,
that's just the starting point for this valuable method.
Expectation values are a form of weighted mean where the weighting
factor can take on many different forms - most often money - so
the method finds many important applications in quality cost and
business cost analysis including:
- Calculate the expected earnings of a simple
game
- Calculate the expected earnings of a business
process (i.e. a more elaborate game)
- Taguchi's Loss Function (i.e. a special game)
- Calculate the threshold value for making a
process change, e.g. a machine adjustment
- Calculate the average sample number for an
acceptance sampling plan or an SPC chart run rule
- Calculate the cost of operating an acceptance
sampling plan or SPC control chart
In addition to using the expectation value method to
characterize a single process, the results from expectation value
calculations can be used to compare variations on a process. For
example, a manufacturing process may include a rework operation,
but how do you know if the rework operation is worth the cost? The
expectation value method can provide the answer. So at this
month's QEN meeting we will review the method of expectation
value, consider its application in some simple games and business
problems, and then look at other applications of the method in
quality cost analysis.
Functional Applications of Machine Learning presented by Keith
Fritz, in person at ASM International (reservation required), or
by Zoom, 7:30-9:00AM, 8 July 2022
Machine Learning (ML), Artificial Intelligence (AI), and
Integrated Computational Materials Engineering (ICME) cover a
broad ranges of topics and applications. This presentation will
focus on the practical real-world applications where these tools
have been applied. Topics include:
- Algorithms to validate test data
- Simulation of manufacturing conditions to investigate defects
- How is machine learning different from traditional six sigma?
- Using ICME to increase material performance
- Resources for further education
Keith Fritz is a Metallurgist from University of Wisconsin and a
GE trained Six Sigma Black Belt. He has held multiple technical
roles at PCC, General Motors, ASM International, and QuesTek
Innovations. He has a passion for the application of Machine
Learning, Data Science, and Integrated Computational Materials
Engineering for solving real world engineering and quality
problems.
The Life Cycle of an SPC Control Chart, 10 June 2022, 7:30-9:00AM, by
Zoom
When Walter Shewhart invented control charts a century ago,
they were constructed using rocks and sticks (aka pencils and
paper). Today SPC software is ubiquitous; however, many people who
practice SPC today don't realize that the time, effort, and cost
limiting considerations that Shewhart intended for the SPC charts
of his day are easily violated by improperly used SPC software. At
this month's QEN meeting we will discuss the design and
administration of SPC charts as intended by Shewhart and what
revisions to his intentions are required today when SPC is
implemented in software.
Taguchi's Loss Function and the S-Double-Bar ($) Chart, 7:30-9:00AM, 13 May 2022, by Zoom
Most of us were taught the goalpost model of interpreting
specification limits: Any units that fall between the
specification limits (aka the goalposts) are good and any units
that fall outside of the specification limits are bad. Taguchi
showed, with his loss function, that real processes aren't that
simple and that a different model for interpreting lost value
(i.e. the cost of units out-of-spec) is required. At this month's
QEN meeting we will discuss Taguchi's Loss Function, its
implications for process improvement using Statistical Process
Control (SPC) and Design of Experiment (DOE) methods, and we will
design a Loss Function-based S-Double-Bar ($) Chart that can be
used to supplement the usual SPC X-bar and R charts.
Misuse of Two-Sample T Tests To Analyze Two-way and Multi-way
Classification Designs, 8 April 2022, 7:30-9:00AM, by Zoom
I have recently seen a flurry of incidents when people have
inappropriately used a two-sample t test to analyze data from a
two-way or multi-way classification design. There is a close
relationship between the two-sample t test, one-way ANOVA, and
two-way or multi-way ANOVA analyses so the cause for such
confusion about the choice of analysis method can be understood.
In fact, under some special circumstances the two-sample t test
may reproduce the results from those other analysis methods;
however, there are circumstances under which the two-sample t test
would be the wrong choice and one of the other methods is
required. We'll talk about this relationship at this month's
meeting and we will look at examples of when there is agreement
and disagreement between the different analysis methods.
Use Operating Characteristic (OC) Curves to Interpret Sampling
Plan Performance, 7:30-9:00AM,
11 March 2022, by Zoom
Acceptance sampling plans, of both the attribute and
variable type, are workhorse methods in quality engineering. We
often pick a plan to meet one or two stated requirements, but we
may not look beyond the plan's sample size and acceptance
criterion to see its detailed performance. The best way to do
that, and to compare the performance of sampling plans to each
other, is to construct their operating characteristic (OC) curves
- a plot of the probability of accepting a lot as a function of
its proportion defective. At this month's meeting we will discuss
how to create the OC curves for attribute and variable sampling
plans, how to interpret them, and how to use them to compare the
performance of two or more competing sampling plans.
The Role of Two-level Factorial, Fractional Factorial, and
Responses Surface Designs in a Program of Designed Experiments,
7:30-9:00AM, 11 February 2022, by Zoom
At our December 10th meeting we introduced the two-level factorial
experiment designs and at our January 14th meeting we extended
them to the fractional factorial and response surface designs. At
the end of the January 14th meeting we mentioned the role that all
three types of designs play in a product or process development
program. It's unusual that we ever run a development program that
involves only a single experiment. Usually a series of experiments
are required, of different design types to match the specific
learning needs of each stage in the development program. Now that
we have some knowledge of the two-level factorials and their
extended designs, let's use our next QEN meeting to discuss how
those various designs are used through the stages of a development
program and how they can put you ahead of schedule and under
budget.
Extending the Two-Level Factorial Designs to Fractional
Factorial and Response Surface Designs, 7:30-9:00AM, 14 January 2022, by Zoom
This session is a follow up to our last (December 10)
session when we discussed the basics of the two-level factorial
experiment designs. In those designs all of the study variables
(i.e the independent variables), whether they are of attribute or
variable type, appear at only two levels. For example, in the
paper helicopter experiment that we considered, the 2^3 experiment
design had three variables: blade length (short/long), blade width
(narrow/wide), and paper clip (without/with). We used ANOVA (or
regression - they do the same thing) to test for response
differences between each variable's two levels. This simplicity
makes these experiment designs tremendously useful - you could
base a whole career on them. However, quite soon you will run into
two of their limitations:
- When the number of number of study variables
becomes large the number of runs required to build the design
quickly becomes impractical
- When the study variables are quantitative and
there is significant curvature in the y versus x relationship
a linear model is inappropriate
These limitations can be overcome by simple
modifications to the two-level factorial designs - by the
fractional factorial designs in the first situation and by
response surface designs in the second situation. We will look at
these designs in this month's session.
An Introduction to Two-Level Factorial Experiment Designs,
7:30-9:00AM, 10 December 2021, by Zoom
Design of Experiments (DOE) is a very broad topic and textbooks
and courses on the topic can be very intimidating; however, the
most basic concepts of the design and analysis of designed
experiments are embodied in the very simple two-level factorial
designs. In these experiments all process input variables (PIV),
whether they are of attribute or variable type, appear at only two
levels. This greatly simplifies the DOE analysis and
interpretation because both ANOVA and regression methods,
whichever you prefer, may be used. At this month's QEN meeting we
will consider these two-level factorial designs, we'll look at
their application to a simple classroom exercise (paper
helicopters), and we'll talk about their role at the core of the
fractional factorial and response surface designs. This topic may
be extended to more sessions if there is sufficient interest.
Design of SPC Chart Run Rules, 7:30-9:00AM, 12 November 2021,
by Zoom
In many circumstances, Walter Shewhart's Western Electric Rules
for out-of-control patterns on SPC charts are safe and sufficient;
however, Shewhart didn't anticipate that SPC charts could become
automated. SPC automation presents the potentially serious problem
of excessive Type 1 errors (false alarms) if too many run rules
are used, especially when too many charts are kept. This risk can
be managed by proper run rule design that takes into account the
number of rules and the number of charts. At this month's QEN
meeting we will discuss Shewhart's design criteria for run rules,
their performance in the context of Shewhart's era, and how the
rules can be modified to be save in the modern context of
automated SPC charts.
The SPC Between/Within Subgroups Chart, 7:30-9:00AM, 8 October 2021, by Zoom
At this month's QEN meeting will will discuss SPC
Between/Within Subgroups Charts and the associated process
capability analysis.
The traditional SPC x-bar and R charts track changes in the mean
on the x-bar chart and changes in the within-subgroup variation on
the R chart. The x-bar chart's control limits are determined from
R-bar from the R chart. The x-bar and R charts are very effective
when out-of-control events on the x-bar chart are limited to
spurious special causes; however, they can fail when the
within-subgroup variation indicated on the R chart does not
correctly capture the range of variation seen on the x-bar chart.
Some common situations in which this problem occurs is when the
subgroups come from different lots with no production continuity
between them or the subgroups means are slowly drifting. This
problem is addressed in an alternative SPC chart called a
Between/Within chart. Three charts are maintained in the
Between/Within chart method: the x-bar chart with modified control
limits, a moving range chart of subgroup means, and the original R
chart. This three-chart set allows the between-subgroup variation
that appears on the standard x-bar chart to be partitioned into
two components: a between-subgroups long term component and a
between-subgroups short term component. The benefit of this
approach is that it provides a more useful set of control charts
that correctly account for the different types of variation in the
process and it can be used to calculate a more accurate set of
process capability statistics that indicate the true capability of
the process.
MINITAB's Graph Builder Tool, 7:30-9:00AM, 10
September 2021, by Zoom
Finding the right graphical presentation method for a data set can
be crucial to conveying the correct interpretation of the data to
your audience; however, finding that perfect graph can be a
challenge. For example, given a single sample of measurement values
we could construct a dotplot, boxplot, histogram, stem-and-leaf
plot, normal plot, run chart, individual value plot, interval plot,
and I'm sure there are others. The magnitude of the problem grows
when we add classifying variables or dependent (e.g. y(x))
variables. To help us along on our searches for these perfect graphs
MINITAB's new release (V. 20.3) includes a new Graph Builder
tool in the Graphs menu. The Graph Builder tool
provides a quick way of constructing many different graphs from the
same data set so that we can inspect them to choose our favorite.
We'll take a look at the MINITAB Graph Builder tool at this
month's QEN meeting.
Using Jitter to Address a Coarse Measurement
Scale When Assessing Normality, 7:30-9:00AM, 13 August 2021 by
Zoom
One of the most common assumptions we check in the many different
statistical analyses that we perform is the assumption of normality.
Normality tests appear in ordinary confidence intervals and
hypothesis tests, acceptance sampling for variables, SPC, process
capability, gage R&R studies, DOE, reliability, and many other
areas. The two most commonly used normality test methods are the
normal probability plot and the Anderson-Darling Test. The
subjective and quantitative nature of the two methods, respectively,
complement each other nicely, but the Anderson-Darling test can be
susceptible to some errors that can be easy to identify and mitigate
if you're paying attention. One of these error situations is when
the measurement data are collected on a coarse measurement scale -
especially when all of the observations fall into only a few
measurement value bins. In many cases the compromised validity of
the Anderson-Darling test can be salvaged by the use of jittering
the original data. At this month's meeting we'll discuss this case,
when the use of jitter is appropriate, how to implement jitter, and
how to report the results.
Crafting Hypotheses, 7:30-9:00AM, 11 June 2021 by
Zoom
The issues surrounding statistical methods can present a bewildering
array of choices to statistical novices. One of the most crucial
topics - and the among the most difficult to learn - is how to craft
the hypotheses for a hypothesis test. If you do this step
incorrectly you can get into all kinds of trouble and the only way
to learn it is either by making lots of mistakes (and getting
caught) or by careful study. The careful study route is a lot less
traumatic, so this month we will talk about the many issues that
have to be considered when crafting hypotheses including:
- What distribution parameter to test?
- What context: one sample, two samples, paired samples, or many
samples?
- What type of data: attribute or variable?
- What distribution to use?
- What analysis method to use: confidence interval or hypothesis
test?
- What type of test to use: significance test or equivalence
test?
- How to phrase the null and alternate hypotheses?
- What decision criterion to use?
Improving the Quality of Your Graphical
Presentations, 7:30-9:00AM, 14 May 2021, by Zoom
I recently suffered through a presentation that included a series of
horrendous graphs. Axis labels were missing, measurement units were
missing, the font size of numbers on the axis scales were too small
to read, legends were missing or made no sense, and the charts were
filled with clutter and special effects that were distracting and
added no value. I learned long ago (it was beaten into me) to make
my graphs complete and as lean as possible but no leaner than
necessary. (I have a great story for you on this theme.) Where do
you learn what distinguishes good and bad graphical displays? It
comes with experience but if you want to learn faster the authority
of graphical data presentation best practices is Edward Tufte who
published a famous and beautiful collection of books on the topic (https://www.edwardtufte.com/tufte/books_vdqi).
We'll discuss some of these best and worst practices in this month's
meeting.
Gage R&R Studies With More Variables Than
Operator and Part, 7:30-9:00AM, 9 April 2021, by Zoom
At last month's QEN meeting we talked about MINITAB's Type 1 Gage
Study and Gage Linearity and Bias Study in relation to the classic
Gage R&R Study operator by part crossed design. It came up in
the conversation that it's possible to have more variables in a gage
R&R study than just operator and part. For example, you might
also consider:
- Using different measurement instruments
- Measuring with versus without a jig or fixture to hold the
part or the instrument
- Comparing collections of operators by shifts or skills (e.g.
shop floor operators versus metrology lab technicians)
- Measuring in different environments (production floor versus
clean room)
And many other study variables are possible. MINITAB provides
support for these modified GR&R study designs in its Stat>
Quality Tools> Gage Study> Gage R&R Study (Expanded)
menu but the analysis can also be done using Stat> ANOVA>
General Linear Model. We'll look at some of these more
complicated experiment designs and analysis tools at this month's
meeting.
Comparing MINITAB's Three Gage Study Methods:
Type 1 Study, Linearity and Bias Study, and GR&R Study,
7:30-9:00AM, 12 March 2021, by Zoom
The gage R&R study operator-by-part crossed design, implemented
in MINITAB's Stat> Quality Tools> Gage Study> Gage
R&R Study (Crossed) method, is by far the most-used gage
study method; however, MINITAB also provides two other gage study
methods: the Type 1 Gage Study and the Gage Linearity and Bias
Study. The capabilities of these three methods have some overlap but
they each have some unique features. At this month's QEN meeting we
will compare these three gage study methods and discuss what
conditions would indicate a preference for one method over the
others.
Part Selection for Gage R&R Studies,
7:30-9:00AM, 12 February 2021, by Zoom
Many people give little thought to part selection for their GR&R
studies; however, the choice of parts can have a huge impact on the
results and usefulness for these studies. As one example: the choice
of parts for a study must be matched to the measurement intent.
Suppose that a process produces parts that have a much tighter
distribution than the spec limits allow (i.e. the process capability
is excellent). If the purpose of the intended measurement is to
support process capability claims then drawing a random sample of
parts from that process would be appropriate; however, if the
purpose of the measurement is to check parts against the spec limits
then a random sample of parts from the process probably won't span
the entire range of the spec and parts with more variability would
be appropriate. There are many other situations that must be
considered when choosing parts for a gage study. We'll talk about
them at this month's meeting.
ASQ Certification, 7:30-9:00AM, 15 January 2021, by Zoom
Within your own company your managers and peers probably have a good
idea of your quality engineering skill set but if you want to
reinforce their opinions or obtain a credential that is known and
valued outside of your company then you should consider obtaining
one of the American Society for Quality's (ASQ) certifications. ASQ
offers 18 different certifications in the quality field
(https://asq.org/cert/catalog). Some of the certifications that will
be of interest to the QMN and QEN audience are:
- Certified Quality Engineer
- Certified Six Sigma Black Belt
- Certified Reliability Engineer
- Certified Quality Auditor
- Certified Quality Inspector
- Certified Quality Technician
At this month's QEN meeting we will talk about the types of
certifications that ASQ offers, their technical scope, the
requirements for applying for certification, how to prepare for the
exam, and the general value of holding these certifications.
Free Software!, 7:30-9:00AM, Friday, 11 December
2020, by Zoom
Everybody loves free stuff! This is a topic that we do periodically
that's always a lot of fun and everyone leaves finding something
that they need or at least want to try out. I've posted my four page
list of free software that you can check out here (Warning: it hasn't
been updated in a while) but plan to come with your own suggestions.
Tests for Proportions or Fractions Defective, 7:30-9:00AM,
13 November 2020, by Zoom
I was in a meeting recently with a customer where the topic of
discussion was the possibility of a difference between the defective
rates of two product assembly processes. When both processes were
running well they were expected to have similar defective rates but
one of the processes was more sensitive to environmental conditions
and could go bad more easily. Recent experience suggested that the
defective rates of the two processes had diverged so a simple
experiment was performed by collecting 20 random units from each
process and inspecting them for defective units. There were no
defectives found in the sample from the robust process and there
were three defectives found in the sample from the weaker process.
At first glance this result feels conclusive - there must be a
difference in the defective rates between the two processes;
however, a formal statistical test (Fisher's Exact test) indicates
that there is a high probability that the observed result could have
been obtained by random chance. This case is an example of a
statistical test for two proportions. At this week's QEN meeting we
will discuss this and similar situations:
- Test for one proportion: Is there evidence that a process has
drifted away from its known historical baseline defective rate?
- Test for two proportions: Is there evidence for a difference
between the defective rates of two processes?
- Test for many proportions: Do many treatment groups share a
common defective rate or is there evidence for differences in
the defective rates between groups?
Two Types of Missing Values in Data Sets: Data
Truncation and Data Censoring, 7:30-9:00AM, 9 October 2020, by
Zoom
At last month's QEN meeting we discussed the use of mathematical
transformations to convert nonnormal responses to normal so that
classical statistical tests and analysis methods can be used. During
that discussion we mentioned the possibility of encountering
incomplete data sets; that is, data sets that have some observations
that are missing. We're not talking about the common case of
observations missing-at-random (MAR) here. We're talking about
observations that are missing-with-cause (MWC). There are several
kinds of MWC situations but this month we'll discuss two of the most
common ones:
- Truncation: Some observations are selectively omitted from the
data set; for example, observations that fall outside of
specification limits
- Censoring: Observations from a product life study that are
missing because the life test was suspended before all units
failed
Variable Transformations for Nonnormal Data, 7:30-9:00AM,
11 September 2020, by Zoom
I recently heard from a customer who was struggling to analyze and
present data which had a number of outliers with large values in the
data set. She was trying to make the case that those observations
were different from the others so that they could be omitted from
the analysis; however, after omitting them and reanalyzing the data
there were even more observations that looked like outliers. This
turned out to be the classic case of the need for a variable
transform - specifically a log transform. This is a common
occurrence in statistical analysis - that a response requires a
transformation - so the method is used everywhere including
inferential methods, gage studies, process capability studies,
designed experiments, statistical process control, and many other
situations. With some practice and experience you can even learn to
recognize when a transformation will be required and which
transformation will do the job given the fundamental first
principles of the process that produced the response.
Use An Input-Process-Output Diagram to Document
the Variables in Your Process, 7:30-9:00AM, 14 August 2020, by
Zoom
We all use the well known Cause and Effect Diagram (aka Fishbone or
Ishikawa diagram) for documenting the factors that affect a process.
Recall that in the absence of specific categories for organizing the
bones on the fishbone diagram we use the default ones: Manpower,
Methods, Machine, Materials, and Environment. The IPO diagram's
structure is taken from the fishbone diagram; the process name is
identified in the middle of the diagram, the process inputs are
presented in fishbone structure to the left, and the process outputs
are presented in fishbone structure to the right. I (Paul) have
found the IPO diagram to be invaluable for quickly communicating the
process input and output variables to a new team member or to a
manager who underestimates the complexity of the system. There are
many ways to build a fishbone diagram. Note cards on a bulletin
board work great, but we will use the free software package FreeMind
to study the construction of and some examples of IPO diagrams.
Which Interval Do I Need: Confidence, Prediction,
or Tolerance?, 7:30-9:00AM, 10 July 2020, by Zoom
Statistical software like MINITAB can be an amazing tool but it can
also present a bewildering collection of methods that look so much
alike that it can be difficult to determine which ones are
interchangeable and which ones are different. One such collection of
methods is statistical intervals including confidence intervals,
prediction intervals, and tolerance intervals. At this month's QEN
meeting we'll discuss the calculation, use, and interpretation of
each type of statistical interval. If you have any examples or data
you would like to share for the discussion, please send them to me
(Paul) with a short description of what you're trying to do.
Improve the Quality of Your Inspection Results by
Upgrading the Measurement Scale, 7:30-9:00AM, 12 June 2020, by
Zoom
I've seen a recent flurry of customers who were struggling to define
methods to characterize somewhat subjective quality characteristics
in their experiments:
- Tom C - Deviation of a particle size distribution from the
ideal distribution
- Travis D - Severity of machined part defects
- Mark P - Response of test subjects in treatment and control
arms of a clinical trial
- Henry P - Demonstrate the reliability of a device
In each case their first thoughts were to use a binary (pass or
fail) inspection criterion - and that method would work except that
binary responses typically demand very large sample sizes that may
not be practical or possible due to time and resource constraints.
In each case we solved the problem by upgrading the measurement
scale that was used to record the observations to a scale of higher
value/information content resulting in significantly reduced sample
sizes.
The value of individual observations increases according to the
following measurement scale hierarchy: nominal (of which binary is a
special case), ordinal, interval, and ratio. Understanding this
hierarchy presents the opportunity to improve your data collection
processes (e.g. acceptance sampling, SPC, process capability, and
DOE) by replacing low-value observations with observations of higher
value. The higher value observations carry more information than the
lower values ones so sample sizes can be smaller - in some cases
smaller by a factor of 10 to 30. At this month's meeting, we'll
review the hierarchy of measurement scales, discuss the
opportunities and benefits of replacing low-value observations with
high-value observations, and the possibilities for reducing
experimental sample size.
Errors, Mistakes, and
Failures of Measurement Instruments, 8 May 2020, 7:30-9:00AM,
by Zoom
At last month's QEN meeting we discussed considerations in choosing
a measurement instrument to match the requirements of a measurement
task. We discussed the usual issues: range, discrimination,
linearity, repeatability, reproducibility, and measurement goal -
whether the measurement's intent is to determine if parts meet
specification limits or to determine process capability. Carefully
choosing a measurement instrument should always happen at the
beginning of the life cycle of a measurement process but during that
life cycle measurement instruments can fail. Let's spend this
session talking about our experiences with failed measurement
instruments including the conditions that caused the failure, the
impact on the instrument, the time elapsed between when an
instrument failed and when the failure was detected, and the
associated consequences to the business.
Considerations in Choosing a Measurement
Instrument, 10 April 2020, 7:30-9:00AM.
Join the on-line meeting using Zoom here
or from your web browser using meeting ID: 421 185 186 and
password: 022 990.
We had a request at our last meeting to discuss how to choose an
appropriate measurement instrument for an inspection operation. This
question isn't as simple as it sounds. The usual first thought is to
identify candidate instruments by their measurement range and then
to choose the specific instrument using the rule of 10; i.e. that
the measurement instrument's resolution/graduations must be less
then or equal to 1/10th of the part tolerance. That algorithm
provides a starting point; however, it is also necessary to consider
the gage R&R capability of the instrument, its measurement
uncertainty (in the accuracy sense), the part's process capability,
and whether the purpose of the inspection operation is to collect
data to be used to determine process capability or just to provide
pass/fail results relative to specification limits. We'll discuss
all of these issues at our April 10th meeting.
Quality Audit Checklists with Examples, 13 March 2020, 7:30-9:00AM, at GGP
Quality audit checklists are a crucial quality management tool for
processes that are complex and difficult to quantify. At this
month's meeting we will discuss the design, construction, and use of
audit checklists and we'll look at some obscure examples including
1) a checklist for evaluating the use of SPC within an organization,
2) a health and safety checklist, and 3) a checklist for evaluating
quality culture within an organization at the upper management,
middle management, and worker levels. The results from the quality
culture audits are especially fascinating - they provide very clear
indications of healthy and disfunctional quality cultures.
Process Precontrol, 14 February 2020,
7:30-9:00AM, at GGP
At this month's meeting we're going to discuss an
alternative to Statistical Process Control (SPC) called Process
Precontrol.
We spent our last two sessions talking about the design and
operation of SPC charts. SPC works best when we have long production
runs of a single product. SPC can still be used for short production
runs using special Short Run SPC methods but those methods can be
complicated and require a knowledgeable and experienced SPC
practitioner. A simple alternative method to Short Run SPC that can
also be used for long runs is Process Precontrol. Process Precontrol
works by starting in a 100% inspection mode until there is
sufficient evidence that the process is stable and then shifting to
a sampling mode. After entering sampling mode, we draw periodic
samples to assess the current state of the process and either stay
in sampling mode when the data look good or switch back to 100%
inspection mode when the data indicates that the process has gone
out of control.
Statistical Process Control, Part 2, 17 January
2020, 7:30-9:00AM, at GGP
At last month's QEN meeting we started a discussion about the basics
of statistical process control (SPC) including the design and
operation of IMR and x-bar and R charts. This month we'll continue
our discussion of SPC by considering more types of charts and go
into the design and operation of them in more detail. We'll also
talk about sample size, sampling frequency, the risks of using too
many charts at one time, and the life cycle of a control chart.
An Introduction to SPC and Control Charts, 13
December 2019, 7:30-9:00AM, at
GGP
Perhaps the single, most effective and ubiquitous process
improvement method in existence is statistical process control
(SPC). SPC is a foundational tool in the quality engineering tool
set and deserves at least some attention from anyone who deals with
any type of process data and substantial attention from experts
within the organization. At this month's QEN meeting we will look at
some of the motivations and philosophy behind SPC, its role in
quality costs, and the simplest of the control charts - the
individual and moving range (IMR) charts and xbar and R charts. We
can discuss other types of charts and advanced methods in future
meetings.
Testing Data for Normality and What to Do When
They're Not, 8 November 2019, 7:30-9:00AM, at GGP
In our last two meetings on process capability we saw that it was
very important to test process capability data for normality.
Normality testing also has a huge role in many other statistical
methodologies including SPC, acceptance sampling, GR&R studies,
Design of Experiments, reliability, and many more. At this month's
meeting we'll look at some of the most popular methods for testing
data for normality starting with normal probability plots and the
Anderson-Darling test. We'll also look at the use of variable
transforms (such as square roots and logarithms) to transform data
from non-normal to normal and we'll look at related methods for
fitting other distributions that aren't inherently normal.
Process Capability, 11 October 2019, 7:30-9:00AM,
at GGP
At this month's QEN meeting we will continue our discussion of
process capability. We'll review the basic process capability
statistics Cp, Cpk, Pp, and Ppk and their confidence intervals and
how to interpret them. We'll use those observations to develop
sample size guidelines for process capability studies. We'll also
look at methods of assessing distribution shape (the common process
capability statistics require a normal distribution) and the use of
transformations to convert non-normal distributions back to normal
distributions.
Process Capability, 13 September 2019,
7:30-9:00AM, at GGP
At this month's QEN meeting we will take up a discussion of process
capability. We'll review the basic process capability statistics Cp,
Cpk, Pp, and Ppk and then discuss their use, interpretation, and the
conditions required for their validity. We'll start a more advanced
discussion of how to do process capability under complicated
conditions, such as for non-normal distributions, and we'll take up
that topic again at the October meeting.
Design and Analysis of Gage R&R Studies (Part
2), 9 August 2019, 7:30-9:00AM, at GGP
At last month's QEN meeting we started a discussion of the design
and analysis of gage R&R studies. We'll take up the topic again
this month by going into more details of the classic operator by
part crossed experiment, paying particular attention to the number
of and selection of operators, parts, and trials for the study.
We'll also discuss extensions of the classic design including nested
designs, designs with additional study variables (i.e. "expanded"
designs), and studies with attribute responses. We may pick up one
or more of these advanced topics in a third session.
Design and Analysis of Gage R&R Studies (Part
1), 12 July 2019, 7:30-9:00AM, at GGP
Measurement reliability is determined by measurement accuracy which
is established by calibration and measurement precision which is
quantified in a gage repeatability and reproducibility or GR&R
study. If a measurement is both accurate and precise then it may be
appropriate for its intended purpose.
The best known GR&R study design is the classic operator by part
crossed design with 3 operators, 10 parts, and 2 trials. Most
references don't give any guidance about why those numbers are used
but good guidance is presented in books like Design and
Analysis of Gauge R&R Studies by
Burdick, Borror, and Montgomery. At this month's QEN meeting we will
talk about how to choose the number of operators, parts, and trials
for your GR&R studies and we'll also discuss other issues like
randomization and blocking in the experiment design, consequences
for the interpretation of the GR&R study report, and how to
integrate instrument type, measurement procedure, the use or not of
a jig or fixture, and other variables into your GR&R study
design. If we have time, we'll start talking about the analysis and
interpretation of GR&R studies but we'll resume that discussion
in more detail at the next meeting.
A Quality Cost Interpretation for Acceptance Sampling
Plans, 14 June 2019, 7:30-9:00AM, at GGP
At last month's QEN meeting we discussed how to design attribute and
variable sampling plans to control defective rates relative to
specification limits. The design of these plans required us to
specify AQL (acceptable quality level) and RQL (rejectable quality
level) conditions that lead to a unique sample size and acceptance
criterion. Although these methods are well known and easily
understood by quality engineers, the AQL and RQL concepts can be too
abstract for others (especially managers) so an alternate, easier to
understand approach is desired. The solution comes by applying
quality cost methods to the acceptance sampling problem. By
specifying the necessary cost inputs (material and labor cost,
inspection cost, and external failure cost) we can express the
performance of a sampling plan in terms of its net income and cost
of poor quality (COPQ). This approach also allows for
easy-to-understand comparisons between different sampling plans such
as the special cases of no inspection and 100% inspection. Even when
the cost information isn't available for a specific process,
understanding the general behavior of quality cost in acceptance
sampling can provide significant insight into the benefits and risks
of the method.
An Introduction to Acceptance Sampling for
Attributes and Variables, 10 May 2019, 7:30-9:00AM, at GGP
Acceptance sampling in quality control is a huge topic but the
simplest acceptance sampling methods are pretty easy to understand.
In a classic acceptance sampling for attributes (i.e. for pass/fail
inspection) application a single random sample is drawn from a lot
and inspected for defectives. If the number of defectives in the
sample is less than or equal to a critical value, called the
acceptance number, the lot is accepted. If the number of defectives
in the sample is greater than the acceptance number then the lot is
rejected. A similar strategy is used for measurement responses by
comparing the mean of a random sample to a critical acceptance
value.
Attributes and variables sampling plans are usually designed to meet
two input criteria which may be:
1) Provide a high probability of accepting good product and a low
probability of accepting bad product
2) Provide a high probability of accepting good product with a zero
acceptance number sampling plan
3) Provide a low probability of accepting bad product with a zero
acceptance number sampling plan
These plans provide different protections for the manufacturer and
for the consumer so it is crucial to understand what you're getting
when you choose a sampling plan. At this month's QEN meeting we will
discuss the design of simple attributes and variables sampling plans
and we'll talk about some of the issues in setting up and operating
them.
Inaugural Meeting: A Survey of Quality
Engineering Methods, 12 April 2019, 7:30-9:00AM, at GGP
The first QEN meeting will be held on Friday, April 12th, from
7:30-9:00 AM at GGP's location in Newbury Business Park when Paul
Mathews and Rick Ales will present a survey of quality engineering
methods for the purpose of assessing the interests and needs of
participants. Learn about the program and facilitators Paul Mathews
and Rick Ales here. To attend email info@geaugagrowth.com or
register here.
The topics to be discussed are but are not limited to:
- Graphical data presentation methods
- Statistical methods
- Statistical problem solving
- Statistical Process Control (SPC)
- Process Capability Studies (PCS)
- Acceptance Sampling
- Gage Repeatability and Reproducibility (GR&R) Studies
- Geometric Dimensioning and Tolerancing (GD&T)
- Design of Experiments (DoE)
- Reliability
- Statistical software, e.g. MINITAB
- Standards, e.g. ISO 9000, ISO/TS 16949
- ASQ Certification
- Quality auditing
- Six Sigma
- Lean
Return to
MM&B Home Page